Maximizing Income and Reducing Costs through AI-Based Monitoring of Customers
Download Case Study
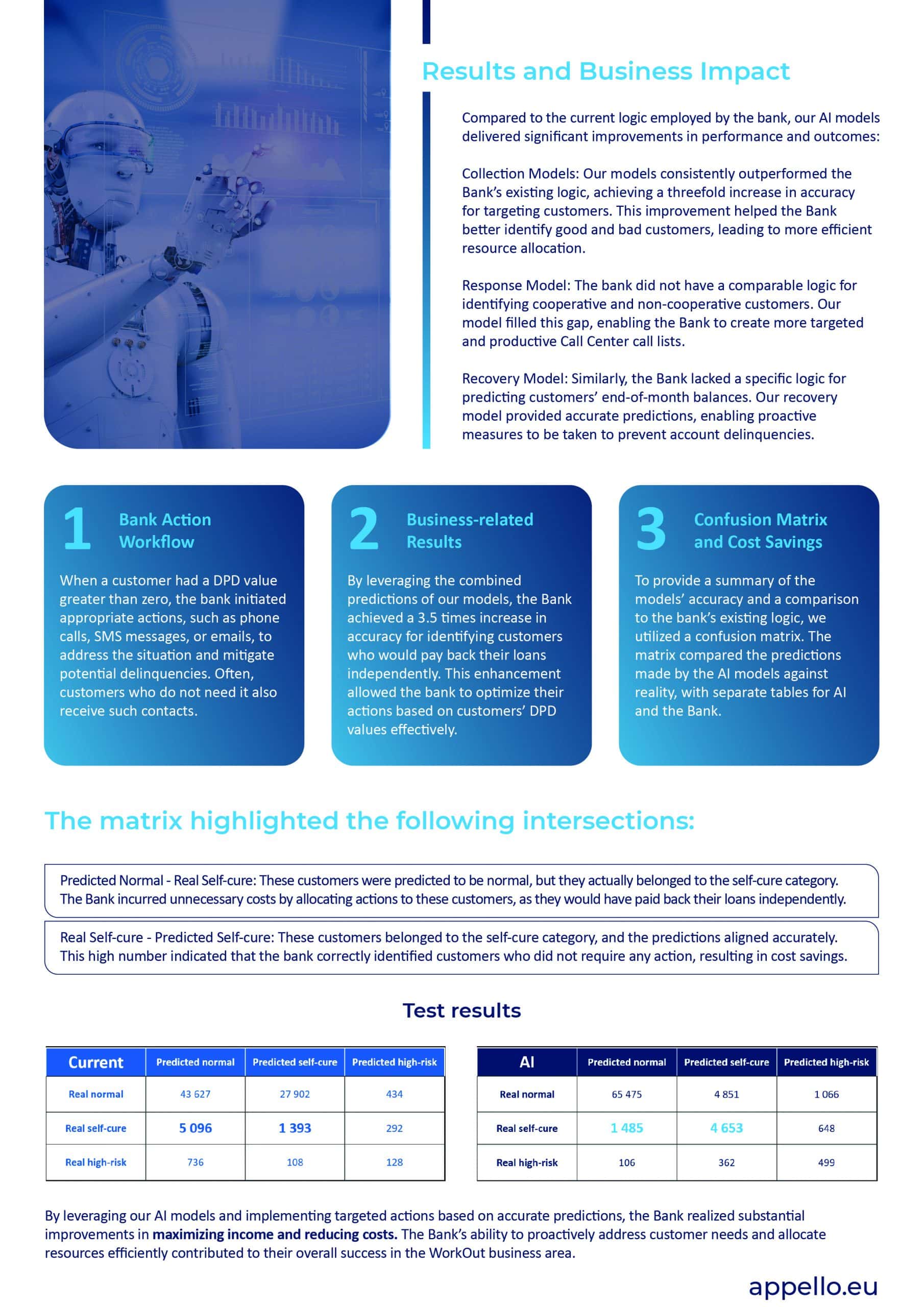
Case Study: Monitoring
Maximizing Income and Reducing Costs through AI-Based Customer Monitoring
Norbert Lukács, Head of AI Division at ApPello summarized his thoughts
and feelings about an interesting and exciting
AI-Based Monitoring Project.
Introduction
The AI-Based Monitoring project started in February 2022 in CEE leading Bank. This Bank aimed to enhance their processes in the WorkOut business area through continuous monitoring. Appello collaborated closely with the bank to achieve their goals of maximizing income and reducing costs.
Approach and Methodology
To address the Bank’s objectives, we identified three key business areas where our AI models could provide valuable insights and support:
- Collection: Our solution involved monitoring Bank B’s clients based on their financial behavior, and targeting good and bad customers efficiently.
- Recovery: Our solution included predicting customers’ balances at the end of the month, enabling proactive measures to be taken for potential account delinquencies.
- Response: This solution aimed to identify cooperative and non-cooperative customers. By filtering non-cooperative customers, it facilitated the creation of a more effective Call Center call list.
We developed a total of five models, each belonging to one of three model families. These models provided actionable signals to Bank for decision-making:
- Collection Models: These models generated signals for Normal Customers, Low-Risk Customers, Self-cure Customers, and High-risk Customers, based on the “Days Past Due” (DPD) values.
- Recovery Model: This model predicted whether a customer’s balance at the end of the month would be positive, negative, or neutral.
- Response Model: This model determined whether a customer was cooperative or non-cooperative, aiding in the creation of targeted call lists for the Call Center.
To ensure the effectiveness of our models, we conducted a comprehensive test phase, executing A-B testing over six months, from August 2022 to January 2023, involving a sample of 70,000 customers. We continuously fine-tuned the models based on monthly results to improve their accuracy.
Results and Business Impact
The AI-Based Monitoring was compared to the current logic employed by the bank, our AI models delivered significant improvements in performance and outcomes:
- Collection Models: Our models consistently outperformed the Bank’s existing logic, achieving a threefold increase in accuracy for targeting customers. This improvement helped the Bank better identify good and bad customers, leading to more efficient resource allocation.
- Response Model: The bank did not have a comparable logic for identifying cooperative and non-cooperative customers. Our model filled this gap, enabling the Bank to create more targeted and productive Call Center call lists.
- Recovery Model: Similarly, the bank lacked a specific logic for predicting customers’ end-of-month balances. Our recovery model provided accurate predictions, enabling proactive measures to be taken to prevent account delinquencies.
Bank Action Workflow
When a customer had a DPD value greater than zero, the bank initiated appropriate actions, such as phone calls, SMS messages, or emails, to address the situation and mitigate potential delinquencies. Often, customers who do not need it also receive such contacts.
Business-related Results
By leveraging the combined predictions of our models, the Bank achieved a 3.5 times increase in accuracy for identifying customers who would pay back their loans independently. This enhancement allowed the bank to optimize their actions based on customers’ DPD values effectively.
Confusion Matrix and Cost Savings
To provide a summary of the models’ accuracy and a comparison to the bank’s existing logic, we utilized a confusion matrix within the AI-Based Monitoring. The matrix compared the predictions made by the AI models against reality, with separate tables for AI and the Bank.
The matrix highlighted the following intersections:
- Predicted Normal – Real Self-cure: These customers were predicted to be normal, but they actually belonged to the self-cure category. The Bank incurred unnecessary costs by allocating actions to these customers, as they would have paid back their loans independently.
- Real Self-cure – Predicted Self-cure: These customers belonged to the self-cure category, and the predictions aligned accurately. This high number indicated that the bank correctly identified customers who did not require any action, resulting in cost savings.
Test result
By leveraging our AI models and implementing targeted actions based on accurate predictions, the Bank realized substantial improvements in maximizing income and reducing costs by AI-Based Monitoring. The Bank’s ability to proactively address customer needs and allocate resources efficiently contributed to their overall success in the WorkOut business area.